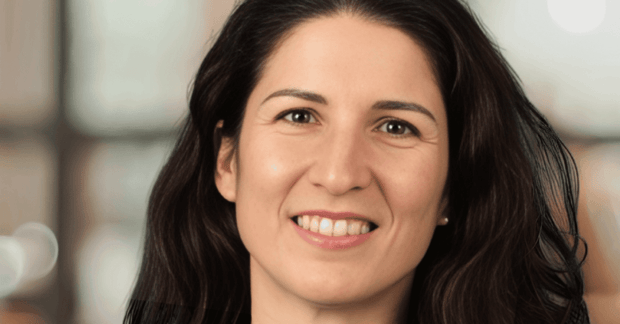
AI governance is an essential process for ensuring generative AI achieves the potential envisioned for it. We spoke to Gabriela Mazorra, former Chair of the AI Governance Working Group with AI Forum NZ, about how we can ensure the AI dream doesn't turn into a nightmare.
Artificial intelligence (AI) governance is a simple concept in principle, but much more complicated to enact. With the evolving state of AI regulation, not to mention the ongoing development of the technology itself, knowing what risk management framework to put in place can be challenging.
To understand how to best leverage AI innovation without putting your organization at risk, you need to leverage AI expertise. That's why consulting with AI governance experts is essential for every organization operating in an AI-driven market.
In the next part of this ongoing series, we spoke to AI governance specialist Gabriela Mazorra, former Chair of the AI Governance Working Group with AI Forum NZ. To find out more about what the market is saying about AI, download our AI survey results:
REPORT: SAP LeanIX AI Survey Results 2024
Meet Gabriela Mazorra
Gabriela has 20 years of experience in corporate governance, risk management, project leadership, and helping organizations navigate complex regulations and make ethical decisions. Her interest in artificial intelligence (AI) governance grew from her belief that data can improve or harm lives depending on how AI-driven decision-making systems are built and used.
This belief inspired her to focus on responsible AI, where she chose to specialise in creating practical, flexible frameworks to help organizations plan for, prioritise, and reduce risks. As a former Chair of the AI Governance Working Group with AI Forum NZ, she has helped expand the community and launch key resources, including the AI Governance website and Workshop Essentials, which support organizations of all types and sizes in building responsible AI practices.
She's currently collaborating with the GOVERNANCE⁴ Group, where she leads their AI governance projects to promote responsible AI and strong risk management. She's also a Charter member of Women in AI Governance (WiAIG), which to fosters collaboration, community, and knowledge sharing across the AI landscape.
In addition to her hands-on experience, she holds a master's degree in technological futures focused on data institutions and is certified as an ODI Data Ethics Professional & Facilitator. Her goal is to help organizations achieve long-lasting, responsible change by preparing for risks and engaging stakeholders, enabling safe, ethical growth in the digital age.
Can You Define AI Governance And Its Importance?
"Artificial intelligence (AI) systems come with diverse risks for organizations, as well as at a wider societal level. For organizations, key risks span four areas:
- Business risks: these include biases, potential job displacement, vendor lock-in, and ethical concerns like opaque decision-making and potential discrimination
- Security risks: AI systems are vulnerable to attacks that can compromise their integrity
- Privacy risks: AI systems often handle personal data, raising concerns about data leaks or unauthorized access
- Operational risks: AI systems deployment can be resource-intensive due to the substantial computing power, storage and expertise required
"This has the potential to disrupt operations and affect the reliability of the system. Governance is central to risk management, aligning processes with organizational values, and existing risk practices to foster a culture of responsible AI use.
"To manage these risks, organizations should establish clear governance frameworks, emphasize responsible AI principles and implement a layered pro-active approach that includes ongoing risks assessment, rigorous testing, clear documentation, and stakeholder consultation. This helps organisation address AI-systems-specific risks effectively and align with ethical and regulatory standards."
How Do You Develop An AI Governance Framework?
"Building a comprehensive artificial intelligence (AI) risk management framework involves clear goal-setting, robust governance, and regular assessments. Organizations need to identify specific AI risks related to their industry and operational environment and set up roles and governance structures for ongoing oversight.
"Good data governance and model oversight are key to managing risks throughout the AI lifecycle. High-quality, well-documented data minimizes biases and inaccuracies. Techniques like differential privacy help protect data, while continuous monitoring for model drift keeps predictions accurate and fair.
"Effective risk mitigation includes creating response plans for potential AI failures and implementing real-time monitoring to detect issues early. Additionally, keeping open channels and establishing user feedback mechanisms, such as those used on social media platforms to report offensive content, can flag algorithmic issues quickly, keeping AI tools safe and trustworthy for end-users."
How Can Organizations Identify AI Bias?
"The process for identifying and mitigating biases in artificial intelligence (AI) models is complex as bias is not a technical issue, but a reflection of human judgement and societal values. Understanding these challenges is the first step for organizations to take a multi-layered approach that hinges on three core practices:
- Diverse and representative data
- Thoughtful algorithm design
- Strong team dynamics backed by targeted training
"Ensuring the selected dataset reflects a diverse population is crucial, as models trained on limited demographics are prone to biased outputs. Data pre-processing techniques can further reduce biases by preventing the model from learning inappropriate associations.
"For riskier use cases, organisation may want to include human-in-the-loop mechanisms. A diverse, well-trained team is equally essential as different perspectives bring fresh insights, helping to identify and address biases."
How Can You Remain Compliant With AI Regulation?
"Ensuring compliance requires staying updated with evolving AI regulations and standards and integrating these into the AI lifecycle. Depending on the industry and geographic operations of an organisation, this can be time consuming and resource intensive.
"Continuous monitoring and auditing are central to managing AI risks as they enable pro-active identification and mitigation of issues before they escalate, particularly as organizations face increasing scrutiny in today's regulatory environment. These processes might involve:
- Automated checks and tools, like AI Verify open-source extensible toolkit, that enable routine validation against ethical principles
- Regular model retraining and fine-tuning to ensure alignment with current data, as well as monitoring for concept drift or deviations from initial objectives
- Employing challenger models to continuously test the efficacy of preferred models, especially when dealing with riskier AI applications
"Monitoring helps track real-time performance and detect anomalies, while audits are focused on verifying adherence to standards and regulations. Together, they provide transparency and accountability, which builds trust and ensures that AI systems operate as intended and stay aligned with organizational values."
How Do You Manage AI Risk?
"Artificial intelligence impact assessments (AIAs) are a pro-active AI risk management strategy for identifying potential risks and opportunities. Rather than aiming to eliminate risks entirely, AIAs help organizations thoughtfully weigh risks against business objectives, facilitating informed decision-making that aligns AI adoption with corporate values and operational goals.
"Other effective AI risk management strategies include scenario testing, using explainable AI (XAI) tools for transparency, robust model documentation process and the creation of specific AI review governance structures comprised of cross-functional stakeholders to evaluate AI projects and enhance accountability ahead of AI deployment. When an AI system fails or shows bias, having a well-defined incident response plan is crucial, and will include:
- Clear processes that help quickly identify and report issues, especially if they might harm individuals
- Investigating the root causes of the incident and documenting the findings from the outset helps build a detailed understanding of what went wrong, why, and how it can be prevented in the future
- Escalation paths and stakeholder communication protocols to inform affected people or groups and clearly explain what happened
- Post-incident reviews to improve systems and processes based on lessons learned, helping to prevent similar issues in the future.
What Capabilities Should An AI Governance Tool Have ?
"AI risk management benefits from tools and processes that provide visibility and tracking across the model lifecycle, supporting responsible AI practices. Model documentation tools, like Google’s Model Cards or IBM’s FactSheets capture key details about a model’s purpose, limitations, and performance metrics helping stakeholders track risks over time.
"Continuous monitoring tools, such as IBM’s AI Fairness 360 and Microsoft’s Fairlearn, detect biases and fairness issues across demographics, ensuring equitable performance across user groups. Additionally, data drift and model performance monitoring solutions like Fiddler, Arize and Amazon SageMaker provide alerts when anomalies arise, allowing for timely responses.
"The offering of end-to-end governance platforms has grown in recent years as well, including Truera AI (acquired by Snowflake) and Holistic AI, which can help with risk tracking and compliance with ethical and regulatory standards. Explainable AI tools like SHAP and LIME further add transparency and can help address interpretability.
"These are only a few of the tools in the market and all tools come with their own limitations that need to be understood and carefully managed by organizations. Using these tools should be part of a comprehensive strategy, rather than standalone solutions. "
To find out more about what the market is saying about artificial intelligence (AI) governance, download our AI survey results: